183. Ahmed Elsamadisi: The Stories Data Can Tell Us If We Ask The Right Questions
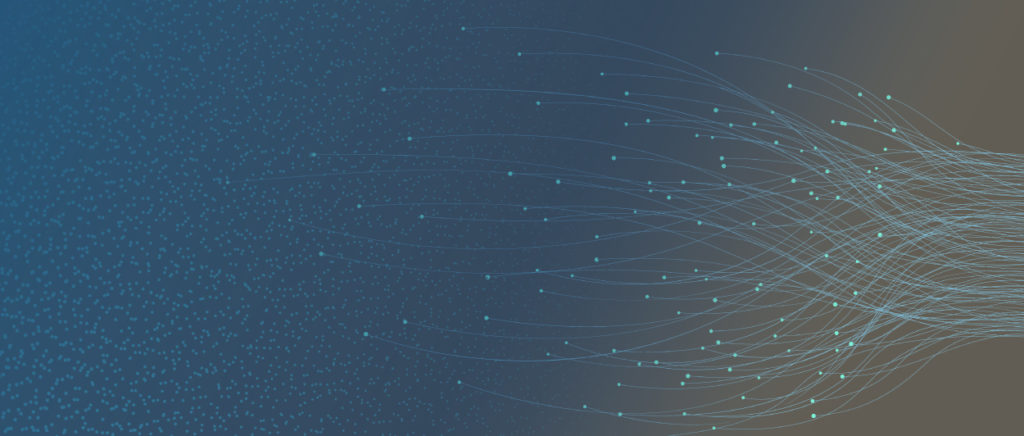
How do companies make decisions? Data certainly don’t make decisions, nor do analytics, nor do the computers they run on. Human begins make decisions — the human factor is crucial. Subjectivism is paramount, even in the age of big data and A.I. The key still lies with the people who are interacting with the data to generate human insights.
Ahmed Elsamadisi is one of the leading data scientists in the world. He’s worked on self-driving cars and nuclear defense and some of the biggest business challenges on earth. He believes that it is the stories we tell from data that drive business success. We are privileged to interview him at Economics For Business podcast, and he gave us a lot of useful advice we can all use every day in managing our businesses.
Key Takeaways and Actionable Insights
The data community has made data and algorithmic analysis far too complex, to the point where it’s no longer useful for business.
The path-dependent route to today’s complex data tables was paved with lots and lots of columns and lots and lots of rows. These data tables are leftovers from the early days of computing SQL language was designed to manipulate these rows and columns. A.I. comes along and can analyze all the possible combinations of data cells. Business executives ask their data departments to generate a lot of these combinations to search for patterns. It often takes a long time, a lot of revisions, and generates no clear answers.
Another aspect of history is the use of dashboards. We tend to design dashboards rather than formulate good business questions. The metrics on dashboards are sometimes useful for operations but they’re often not at all useful for understanding the causal connections between data points. Consequently, different people can interpret them in different ways and there is no consensus as to what they mean and what to do about it.
The purpose of data analytics is to generate good decisions that lead to action.
The entrepreneurial method drives towards D and A: decisions and actions. Analytics should help to formulate the hypotheses on which to base decisions. The problem with complex dashboards and algorithmic pattern recognition is that they often don’t give clear direction on recommended action, especially when the interpretation varies depending on who is doing the interpreting.
Ahmed’s experience is that sharing a numerical dashboard with 10 executives is very likely to result in 10 different interpretations, and the resultant confusion and disagreement freezes action rather than accelerating it.
We need data to tell us stories that we can all rally around.
The most powerful tool for developing consensus around action is narrative — often called storytelling. While 10 dashboard interpretations might lead to 10 different action plans, a single well-told story can align everyone who hears it, understands it, and internalizes it. We heard about the power of narrative in episode #181 (Mises.org/E4B_181) in which Brian Rivera explained the role of storytelling and sensemaking in The Flow System of management, and in episode #152 (Mises.org/E4B_152) where Derek and Laura Cabrera explained the power of aligned mental models for driving business. Stories achieve alignment.
Ahmed Elsamadisi built his service, narrator.ai, to output data analytics in the form of a story. The complexity riddle is removed and replaced with a narrative that all executives, not just data scientists, can understand. Narrator.ai re-integrates data science with the all-important human element of understanding stories.
The way to get data to tell stories is with a conversation.
Ahmed says that the way we ask questions (data queries) is flawed. It’s quite a normal practice to set the A.I. to search the data tables to look for patterns to see if anything interesting emerges. This is what Ahmed calls “lazy hypothesis generation”, which is never going to yield useful actionable insights (yet many big analytics companies are taking in huge customer revenues for just this service). Clients may claim to be making data-driven decisions but that’s mis-characterizing this business behavior, typical though it may be.
Ahmed advises us to think more in terms of a conversation with data. To facilitate this, he has developed a universal data model with just three variables: an entity (such as a customer), an action, and time. Every business question is about a customer taking some action in some time period. The universal data model enables the conversation: what action did the customer take in what period of time, e.g., when did they open the email and what action did they take after opening it. This is not a database query, it’s a more thoughtful question about the customer experience and how to understand it.
Ahmed told us that training customers in this conversational mode of interaction with the universal data model results in a cultural shift in thinking. The conversation can go back and forth in several iterations until the understanding is fully honed. Clients hear the data talking to them through the stories that narrator.ai generates. The have deeper insights and a story to share to form a consensus around the action that the story suggests. Narrator.ai clients have used stories for everything from describing new product specs to updating board decks.
Great conversations with data are based on empathy and thinking about the customer experience.
At Economics For Business, we elevate customer empathy a the most important business skill, in the context of an understanding of customer value as subjective, a good feeling from an enjoyable or satisfying experience.
Ahmed advises us to think in this same way when formulating conversations with data to generate insights. If we think about the customer’s experience, desired and actual, and the actions they take before and after that experience, and the time context of the experience, we’ll do well in formulating good questions. The action component of the universal data model is central to the Austrian deductive method: knowing what people do can help us deduce motivation and expectation. Knowing what they did next can shed light on the ends they had in mind. Actions like opening e-mails or repeat buying are also revealing of intent and expectations. The more we converse with the data, the more insight we can gain.
Storytelling with data is another implementation of subjective quantification — with the benefit of enhanced intuition over time.
In episode #176 (Mises.org/E4B_176), Peter Lewin introduced us to the Austrian concept of subjective quantification — turning customers subjective valuations into numbers such as capital value on a balance sheet. We tested the subjective quantification term with Ahmed, and he endorsed it — with a major addition. It’s important to include the dimension of time. If, over time, we have better and better conversations with data and formulate better questions and hypotheses, we’ll get better and better at generating insights. Our intuition will improve. We’ll get a better “feel” for the data. Even our empathy can become more accurate.
Additional Resources
Narrator.ai and its excellent blog, Narrator.ai/blog
“Top Ten Signs You Have A Data Modeling Problem”: Mises.org/E4B_183_Blog
Ahmed Elsamadisi on LinkedIn: Mises.org/E4B_183_LinkedIn
Responses